Automated Vision AI Platform for Anomaly Detection in Manufacturing
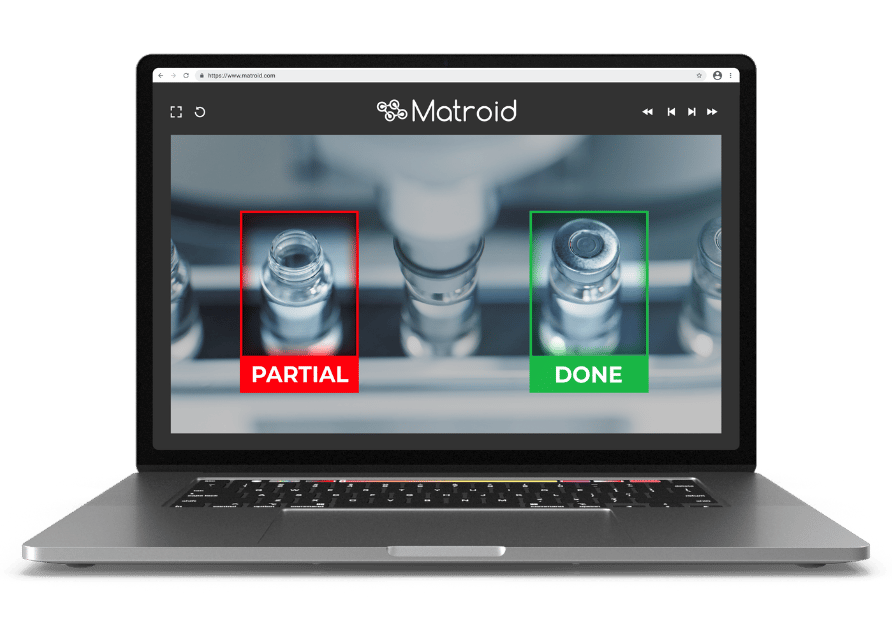
Matroid | March 11th, 2024
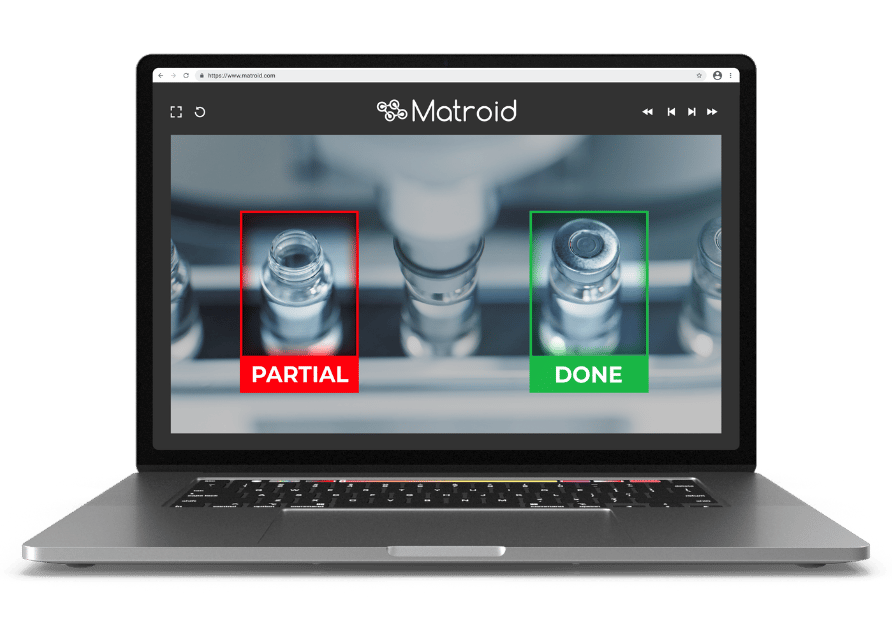
The pursuit of precision and quality is an ongoing challenge in the world of manufacturing. Manufacturing managers and supervisors understand the critical importance of maintaining production integrity and ensuring that every item leaving their factory meets rigorous standards. In the past, reaching this level of precision was a more manual endeavor, relying heavily on human oversight and inspection processes.
Achieving the highest level of quality is greatly aided by advanced technology that can automate the detection of visual anomalies. Matroid’s computer vision solution, using Deep Learning AI techniques, is reshaping the manufacturing landscape by offering improved reliability and control.
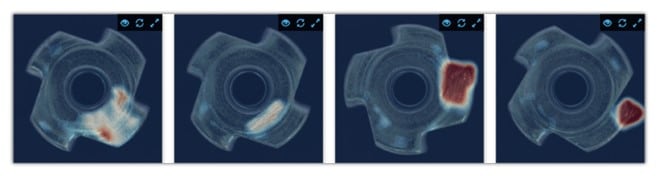
What is AI Visual Anomaly Detection?
Matroid’s visual anomaly detection leverages computer vision, enhanced by Deep Learning, to digitally pinpoint irregularities or defects in the manufacturing process. This approach employs sophisticated algorithms, enabling systems to dynamically learn and adapt to visual data, thus enhancing the efficiency and accuracy of anomaly identification in real-time. Defects identified can encompass a wide range of issues, such as cracks, chips, scratches, dents, discoloration, missing parts, or incorrect assembly, essentially capturing anything that deviates from what is normal.
The scope and application of this technology spans various industries and scenarios – from inspecting raw materials before production to monitoring the quality during manufacturing and conducting final assessments on finished products.
Its versatility and ease in implementation makes it an indispensable tool for anomaly detection in manufacturing and metals.
Benefits of Automated Anomaly Detection Across Industries
Various industries can leverage Matroid’s automated visual anomaly detection. While a food and beverage company might employ the visual detection software to find foreign objects within its products, ensuring quality control, a semiconductor may use it to pinpoint defects in their chips, guaranteeing the integrity of their technology.
Automotive manufacturers need to inspect the paint on their vehicles, to guarantee a smooth finish. Pharmaceutical companies require a meticulous inspection of the packaging of their drugs. Across diverse sectors, computer vision anomaly detection is the common denominator to ensure precision and reliability in different facets of production and quality control.
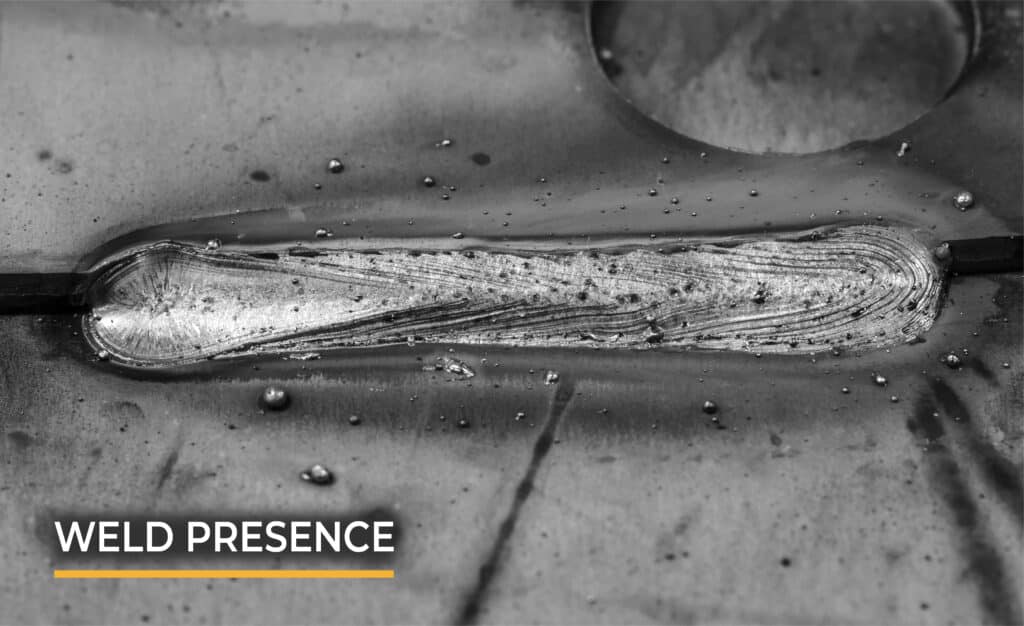
In recent years, automated visual anomaly detection systems have relied more on machine learning algorithms to learn the normal patterns and features present in the data. These algorithms then analyze new visual data and flag or highlight any regions or instances that deviate significantly from the learned normal behavior. However, this kind of detection also has some challenges to seamless implementation in manufacturing settings.
Labeling data is a significant challenge in implementing visual anomaly detection systems. Anomaly detection often falls under the category of unsupervised learning, meaning that algorithms lack labeled data for training. In manufacturing where anomalies are rare, it is difficult to create a thorough dataset for training.
Concept drift presents another hurdle. The normal behavior of a manufacturing process can evolve over time due to factors like equipment wear and tear, alterations in raw materials, or the introduction of new product designs. This makes it harder for anomaly detection algorithms to adapt to changes in the underlying patterns.
The computational expense of anomaly detection in large image or video datasets is also a factor to consider, especially in manufacturing where real-time processing is crucial.
Specific examples of challenges include the variation of illumination in manufacturing environments, as well as occlusion, where one object is partially or completely obstructed from view by another object. Both these issues add complexity and hinder defect pinpointing. The inherent noise in manufacturing environments can also reduce the effectiveness of anomaly detection algorithms.
There is a real need for tailored, robust, and adaptive solutions to address these issues.
How Matroid’s Computer Vision Addresses Detection Challenges
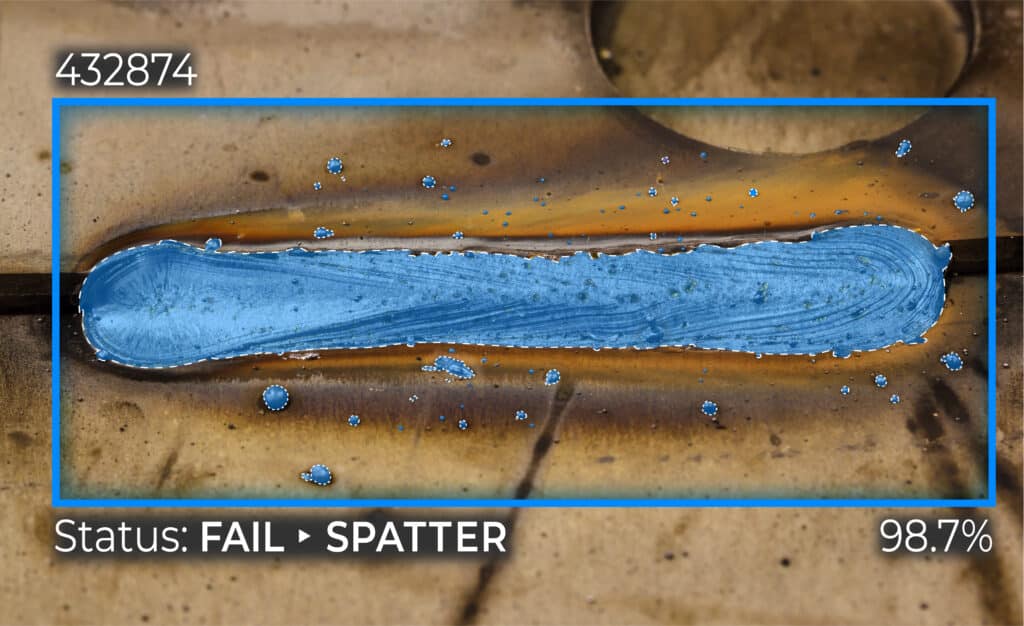
Matroid’s solution effectively addresses these challenges in manufacturing environments. In the manufacturing and other industries, the terms “machine vision” and “computer vision” are often used interchangeably.
However, they are distinct concepts, and technological advancements have facilitated a more powerful method known as Computer Vision.
One key benefit lies in computer vision’s ability to learn from unlabeled data, streamlining the training process for anomaly detection models. This is particularly valuable as it mitigates the hurdles associated with labeling data. Matroid’s solution also mitigates concept drift by adjusting to changes in the normal behavior of a manufacturing process.
Matroid’s computer vision technology stands out for its efficiency, particularly in addressing the computational challenges associated with anomaly detection in manufacturing environments. This capability is crucial in manufacturing, where real time identification of anomalies is important.
The AI enabled defect detection also compensates for illumination variation, ensuring enhanced defect detection under diverse lighting conditions. It can find defects even when they’re partly hidden, offering a complete solution for occlusion issues. Lastly, the technology effectively filters out noise, contributing to improved defect detection in noisy environments.
This multifaceted approach underscores the versatility of Matroid’s software in addressing key challenges associated with anomaly detection in manufacturing settings.
AI Anomaly Detection in Metals Manufacturing
In the metals industry, Matroid’s technology identifies defects in metal castings, such as cracks, pores, and inclusions. It significantly enhances the overall quality of castings while decreasing the number of faulty products reaching customers.
Moreover, its capability to inspect metal surfaces for imperfections like scratches, dents, and discoloration serves as a proactive measure, preventing the integration of flawed metal products into the manufacturing and assembly processes.
The computer vision’s application extends to monitoring other metal processing operations like welding and cutting, enabling early detection and correction of issues – and averting potentially costly damage to equipment and materials.
Lastly, its role in inspecting finished metal products prior to shipment ensures a consistent high quality, effectively reducing returns and warranty claims.
Leading companies, including Service Center Metals (SCM) and JOST International, have embraced the technology, resulting in improved precision, reduced defect-related costs, and elevated production quality, ultimately contributing to the overall profitability of their operations.
Automated Visual Anomaly Detection the Matroid Way
Matroid is redefining the landscape of computer vision and anomaly detection. The mission is to democratize the process by making computer vision readily available – and easily implemented by industry professionals who lack coding experience.
By leveraging machine learning algorithms – and augmenting them with advanced computer vision techniques, Matroid has established a powerful system for efficient and precise anomaly detection.
The advantages of employing Matroid in manufacturing are evident: its remarkable adaptability to the dynamic shifts within manufacturing processes, the efficient utilization of resources for real-time anomaly detection, and the enhanced capability to detect defects under diverse and challenging conditions.
Matroid’s anomaly detection capabilities not only ensure superior product quality but also promote operational efficiency, underscoring the software’s significance as a pioneering solution for robust anomaly detection in the manufacturing industry.
Download Our Free
Step By Step Guide
Building Custom Computer Vision Models with Matroid
Dive into the world of personalized computer vision models with Matroid's comprehensive guide – click to download today